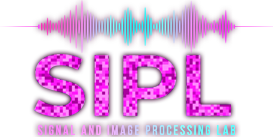
Deep diffractive neural networks have emerged as a promising framework that combines the speed and energy efficiency of optical computing with the power of deep learning. This has opened new possibilities for optical computing suit for machine learning tasks and all-optical sensors. One proposed application of this framework is the design of a diffractive camera that preserves privacy by only imaging target classes while optically erasing all other unwanted classes. In this work, we investigated whether this camera design truly erases the information in unwanted class data.
We used K-NN to achieve up to 94% accuracy in classifying optically erased images. We then used a U-Net to reconstruct the original images from their optically erased counterparts. Our findings revealed that the proposed optical diffractive camera failed to erase important information, suggesting that a different approach may be needed to achieve a truly privacy-preserving diffractive camera.